Policymakers will be will still be aware towards results of the borrowed funds refinancing station into the riches-building opportunities to possess home owners. The brand new money profiles out of Black colored and you will Latina home owners try qualitatively other out-of those of Light and you may Asian property owners, which have a significant display of their riches and you will assets centered from inside the their homes. This skew emphasizes the necessity for enhancing entry to refinancing mortgage, that’s crucial for preserving their homeownership development and you will broadening streams in order to wealth strengthening. While we features underscored the key benefits of deeper mortgage repayment cost and money building, we acknowledge one homeownership is more than just a financial investment. It offers families a feeling of that belong, stability, and you will manage. The low refinancing costs one of lower-income, Black, and you may Latina property owners stress this new pressing dependence on initiatives that promote refinancing while the a strategy to target the newest racial riches pit.
Appendix
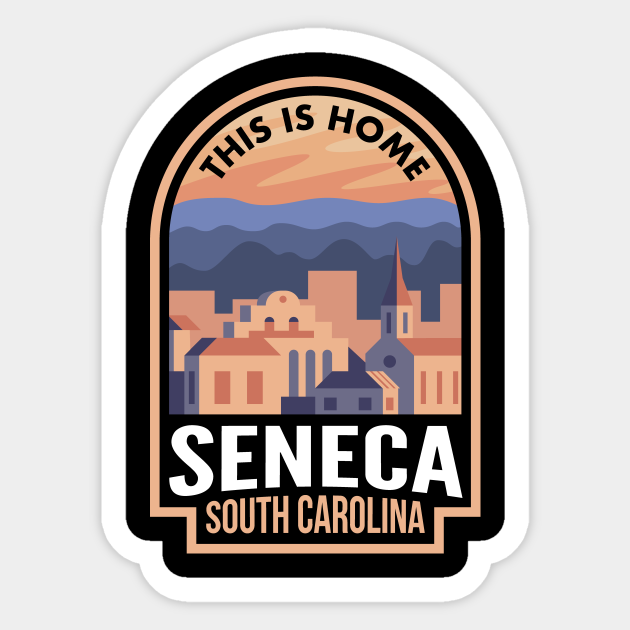
CoreLogic try a professional study aggregator you to focuses on furnishing possessions investigation factors. The fresh CoreLogic action investigation utilized in which analysis will bring across the country coverage off attributes and progression regarding investment interest of this per package. I mutual CoreLogic’s longitudinal package-height studies that have in public areas available HMDA data while the previous study resource does not include information on borrowers’ functions, such as race and you can income. I matched parcel study off CoreLogic on mortgage loans effective anywhere between 2007 and 2021 to help you annual HMDA into the every one of those people decades.
We parsed this new HMDA analysis to add pick and you will refinance mortgages, as the appointed of the loan action style of occupation. After the methodology of Goodman, Bai, and you can Li (2018), i probabilistically coordinated CoreLogic and HMDA study using an array of financing qualities with a high quantities of agreement: census area, origination season, occupancy form of, lien form of, mortgage sort of, financing mission, loan amount, and financial identity. To improve the accuracy of one’s matches rate and reduce this new density of numerous-to-you to definitely suits, we set a similarity tolerance having financing quantities of not any longer than just $step three,000. Requiring a special match toward mortgage number turned-out also limiting and you can did not take into account expected differences when considering the two data offer because of rounding or other types of mistake. This very first stage within our coordinating procedure resulted in the average 60 percent potential match price around the all ages.
To handle differences in lender brands, we made use of the Levenshtein ratio, a commonly used sequence coordinating formula one to steps the resemblance anywhere between strings
The get ranges away from 0 to at least one and you will reflects the quantity regarding changes needed seriously to build several chain equivalent, that have a higher score demonstrating higher sequence similarity. I thought info which have good Levenshtein rating higher than 0.65 realistic matches from inside the bank labels. Which second stage preprocessing procedure yielded the common 41 % sure meets however, will not fully manage new instances of of numerous-to-one suits. From inside the design the very last attempt, we very first picked information having clean that-to-one fits, followed closely by deciding on the meets on the higher Levenshtein rating one of ideas having multiple prospective suits. Some other information had been decrease. The very last try provided 37.5 billion info that have the typical 33 % book suits rate around the all of the ages.
We conducted multiple recognition tests to verify the precision your decide to try suits. I at random chose trials and you can by hand verified the fresh new fits overall performance. We including did cross-checks towards the lender and MSA distributions ranging from the try and Huntsville loans full HMDA dataset, and this showed large interaction among them. On the other hand, we utilized a preventing solution to gauge the sensitivity in our leads to our liking on large Levenshtein get by randomly replacing chosen suits which have choice suits whenever numerous choice was offered. In the long run, we accomplished next awareness tests by varying amount borrowed distinction and you will Levenshtein ratio thresholds, and this confirmed the new structure and robustness of your results.